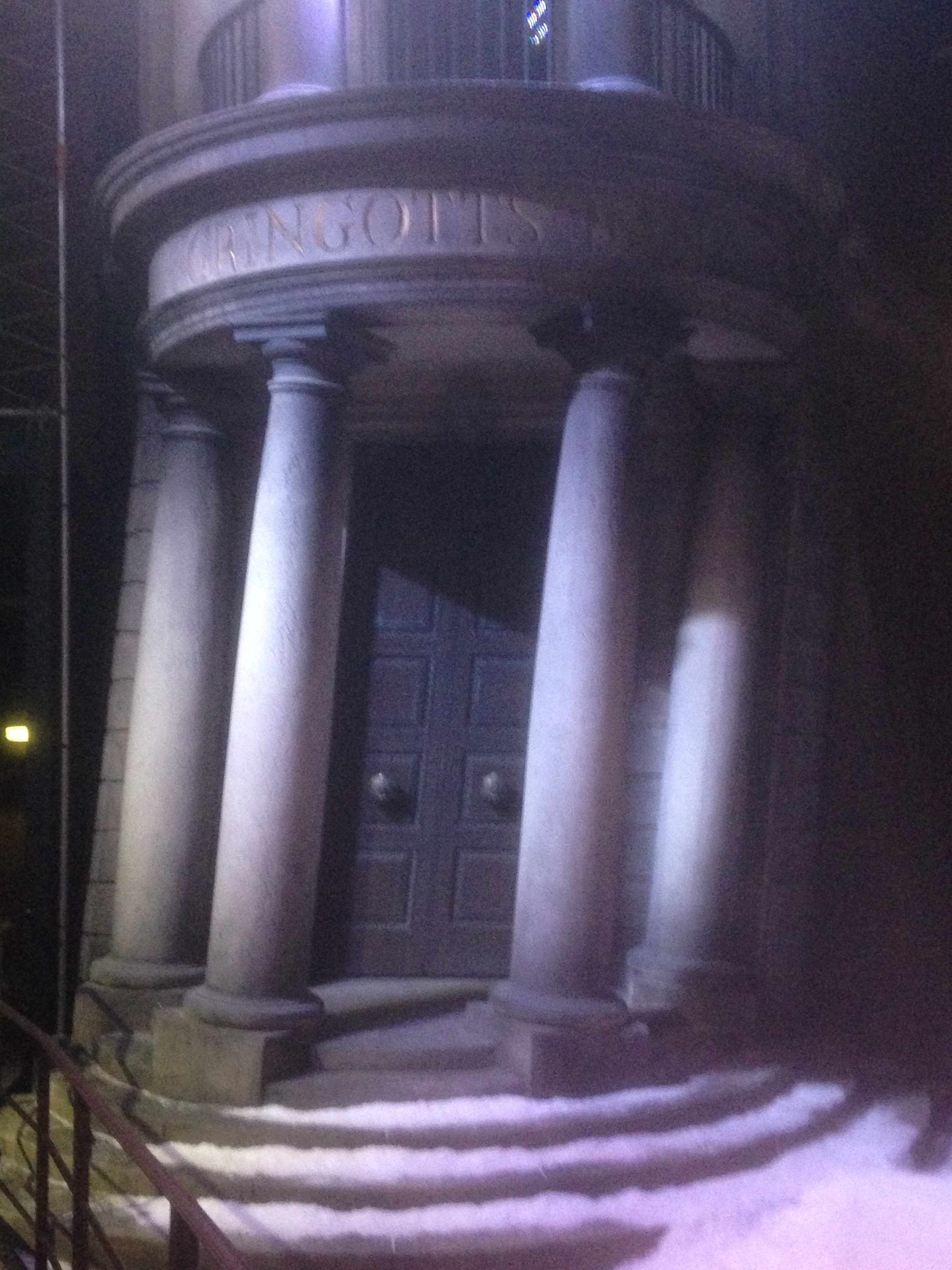
In a world where it is now much easier for consumers and businesses to switch banks, one reason that so few people do switch is that trust in banks still typically outweighs the exasperation that is caused by poor service experience.
Of course, what “good” looks like in terms of service excellence needs to be continuously reappraised in order to keep pace with the expectations of digital consumers. Mapping out the optimal “customer experience” across the range of products and services is a great start-point for this, after which you can map out the processes which deliver these products and services and seek to understand where they deliver a great experience and where they fall short.
Process improvements can then be designed to address the problem areas and information can be gathered and analysed to spot and even predict service delivery shortcomings. When you can predict the problem, you can address it efficiently before it occurs, rather than expensively (both in terms of money and brand) after it has happened. Consistently good service experience will lead to increasing loyalty and a positive reputation in the market, enabling the bank to differentiate itself, retain customers and attract new business.
Naturally, the more complex the web of processes, the more likely they are going to be prone to problems. Simplicity is good. We have all observed how the fintechs and challenger banks have sought to create nimble, cost effective infrastructures which large banks, with a deadweight of complex legacy infrastructure, should in theory find it hard to compete with. To their credit, the challengers appear to have been spending more time than traditional banks, in talking to the market and finding out what customers want in terms of product design and levels of service.
At the moment, the challenger banks are, in many cases, testing the market with simple online products, such as fixed term savings accounts, rather than mirroring the full range of services which the traditional banks offer. Personally, I am not holding my breath for a real breakthrough from these banks – recently the mobile-app-only bank in the UK where I had opened a fixed term savings account managed to make my entire account data invisible to me, after pushing an app update on to my iphone. The process to make this data reappear involved a lengthy re-registration process with their contact centre. If these guys are struggling with technology at this level, it seems to me it will take time for them to develop a robust multi-service capability.
To be honest, I don’t really care about the type of technology – only how it helps support and deliver excellent service at the right price. A bank needs to use technology effectively to connect its data and its processes together and to streamline its service delivery. It should avoid the temptation to use new technology “because it’s there”, without addressing the root cause of service delivery issues.
Clearly this demands that the bank builds a clear view of what sort of service its customers want, and is able to justify the investment either through cost savings or the ability to cross-sell more products. This cross-selling requires a bank to capture information about its consumers, in market segments and ideally also to make it personal at the level of the individual customer.
A couple of ways to do this are to invest in relationship management and to invest in data analytics. Both have real challenges for a bank.
Again from recent personal experience, I have spent at least ten hours in meetings with the local relationship manager from my traditional UK bank, with an eye-watering amount of mundane form filling but also with some really value-adding observations about my potential needs. Trust was built up, and I began to look forward to each interaction. Then, all of a sudden, I got a call from the bank to say that the relationship manager was leaving and that it would be some months before they appointed a replacement. Back to square one, and a sinking feeling that when I do meet the replacement then it will be time to start going through all those forms again.
So, there is a real challenge for banks to invest in a consistent relationship management service, which includes effective two-way communication with customers.
How about removing the “inconvenience” of relying on staff continuity, and trusting in data analytics instead? There is a lot of research and investment going into predictive data. My sense is that this is probably effective at a “segment” level, but that “predictive” can become inaccurately “presumptuous” when such techniques are applied at the level of the single customer. I don’t (thank goodness) have a personal example of this from the banking sector – setting aside all those offers to move to “premium” credit cards which I don’t want or need and which get filed immediately in the recycling bin. But, given that retail banks are inclined to adopt practices from other retailers, I do worry about what might be coming.
Let me take a recent personal example from a well-known travel booking app that I (used to) use. I was recently meeting a new client whose linked-in profile showed that they, like me, had spent a lot of time in the Far East. I was trying to remember the name of a hotel I had stayed in few years ago. Via the history pages on the travel booking app I found my original booking. Great. Until I started getting a daily bombardment of emails from the travel company, offering to help me book my next Far Eastern trip. I have to say that I had seen this as a great app for making bookings and for getting good discounts. But the nuisance effect of those emails outweighed the value of the service, causing me to unsubscribe and to remove the app from my phone.
I give this as an example of what it might take for me to quickly turn off my relationship with a bank. It is all about the grey area where predictive becomes presumptuous, and where marketing becomes intrusive.
What happens is that the bank would reach a point, where it will start to undermine its core competence of “trust”. Trust is not just about security. It is also about relying on a bank not to waste its customers’ time with presumptuous offers which they don’t need or want. A travel company can get away with such approaches. I don’t believe that a bank can, because its trust premium is a fundamental reason it stays in business.
At the start of this blog I commented on the balance of trust and service experience and its effect on customer retention. I have shown how investment in new services can lead to a requirement to build knowledge which, especially when placing too much reliance on data analytics, can lead to unintended breakdowns of trust. My own recommendation is that banks don’t mess with trust but rather focus on the other side of the equation, and invest in eliminating the exasperation that is caused by weaknesses in service delivery.